The ability to effectively manage leads and prioritize prospects is essential for optimizing success in the fast-paced world of sales. Some leads may slip between the cracks because sales teams sometimes interact with hundreds or even thousands of potential clients. This is where artificial intelligence (AI) comes into play, completely changing how sales teams prioritize and score leads.
The technique of ranking prospective clients (leads) according to their propensity to become paying customers is known as lead scoring. Finding the leads that are most worthy of the sales team's attention at any given moment is the goal of prioritization. This was a manual procedure that relied on limited data, experience, and intuition in the past. But thanks to AI, this procedure has changed into one that is far more accurate, data-driven, and efficient.
We'll look at how AI is changing lead scoring and prioritization in this blog, giving sales teams the resources they need to make quicker, more informed decisions that will ultimately result in higher sales.
The Challenge of Traditional Lead Scoring and Prioritization
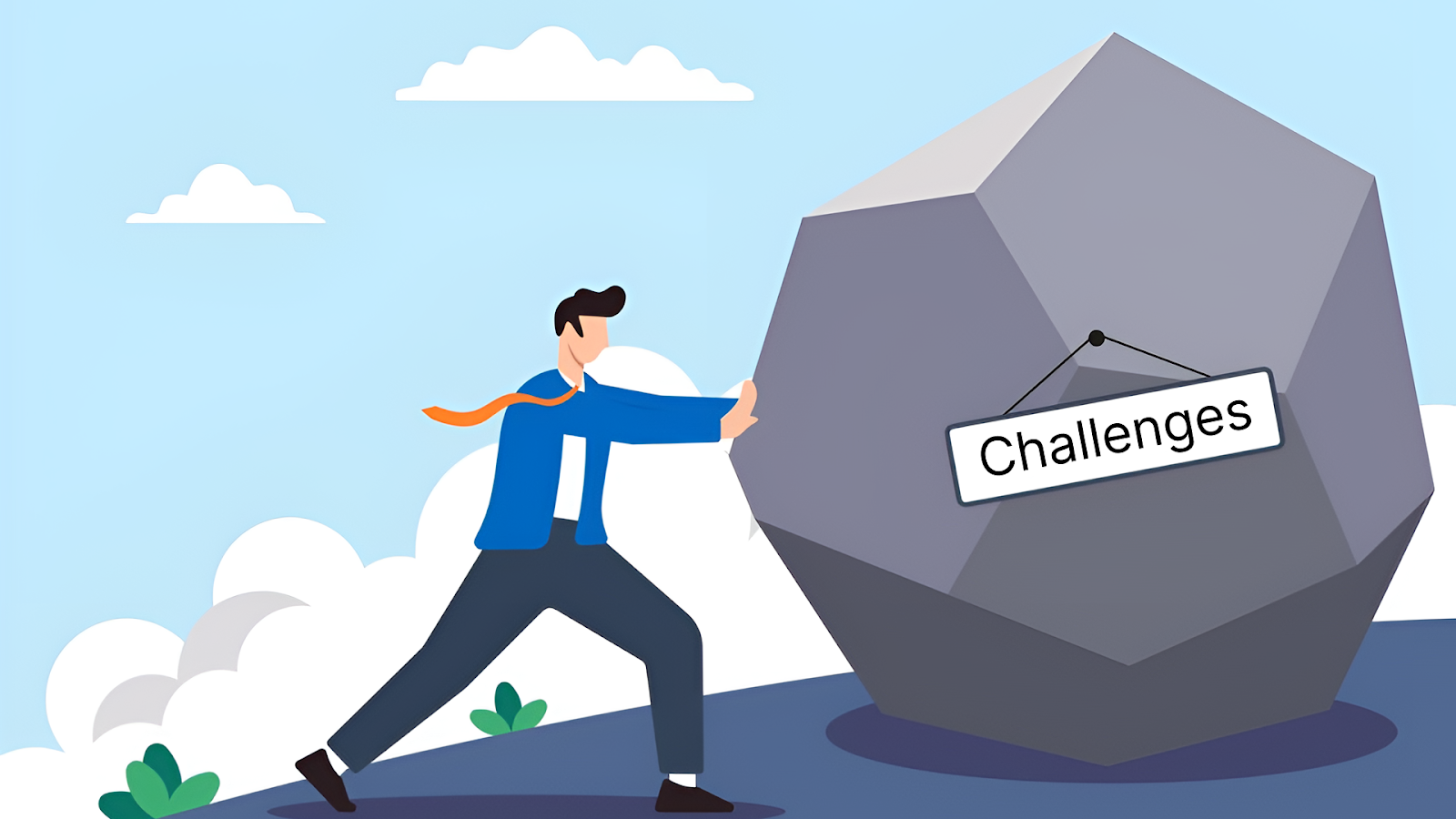
When using traditional lead scoring systems, Sales teams rely on subjective evaluations and historical data. Because salespeople may misunderstand a lead based on prejudices or limited information, this strategy is frequently ineffective and prone to mistakes. Using conventional lead scoring techniques, sales teams have the following difficulties:
- Manual Data Entry and Analysis: A lot of effort is often put in by sales teams manually entering and evaluating data from multiple sources (such as CRM, social media, and website visitors). In addition to taking a long time, this procedure is vulnerable to human mistake.
- Limited Data Points: Traditional methods tend to focus on a limited number of details, such as job title, firm size, and previous interactions, which could not fully represent a lead's potential.
- Subjectivity and Bias: Instead of using unbiased, data-driven insights, salespeople may mistakenly prioritize prospects based on their own preferences or feelings.
- Lack of Real-Time Insights: Sales teams frequently work with out-of-date information, which makes it challenging to make real-time strategy adjustments.
Due to these restrictions, lead scoring is a poor procedure that results in lost opportunities and resource waste.
How AI Improves Lead Scoring and Prioritization
AI handles these issues by using data, machine learning algorithms, and predictive analytics to improve the speed, accuracy, and intelligence of lead scoring and prioritizing. AI can enhance the procedure in the following ways:
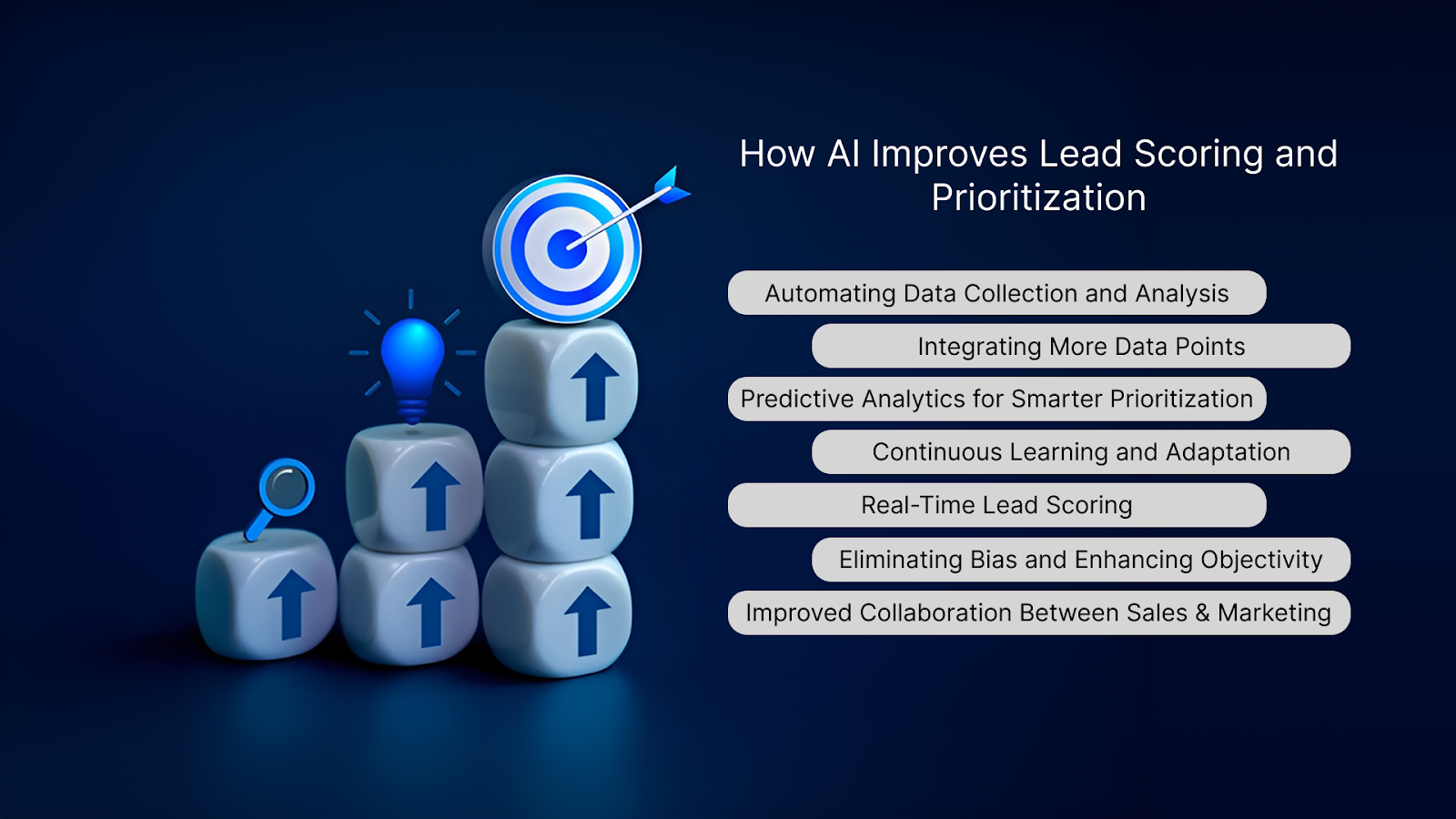
1. Automating Data Collection and Analysis
Data from a range of sources, such as CRM systems, email campaigns, social media, website interactions, and more, can be automatically gathered and analyzed by AI-powered lead scoring systems. AI can collect large amounts of data in real-time, guaranteeing that the lead data is always up-to-date compared to traditional approaches that depend on manual data entering.
AI solutions, for example, can monitor a lead's actions on a business's website, such as page views, downloads, and form submissions, and utilize this information to determine a more precise lead score. After that, the AI system examines the data to find trends and actions—like how often a lead interacts with your content or how long they spend on particular pages—that suggest a lead's tendency to convert.
Why it matters: Time is saved, errors are decreased, and sales teams receive deeper, more accurate insights when data gathering and analysis are automated.
2. Integrating More Data Points
AI is not restricted to traditional data points such as industry, job title, or firm size. A wider variety of data from many sources can be analyzed and integrated by it, including:
- Behavioral data: Details regarding how leads respond to emails, content on websites, or social media.
- Firmographic data: Information unique to a company, including its size, income, or industry.
- Demographic data: Details about the preferences, locations, and employment roles of certain leads.
- Engagement signals: Lead engagement metrics, such as webinar attendance or content downloads, show how actively a lead has interacted with your content.
AI generates a more accurate and detailed picture of each lead by combining all these independent data sets, which results in more trustworthy lead scoring.
Why it matters: Sales teams may focus their efforts on high-potential prospects by using AI to estimate which leads are most likely to convert, which improves with the amount of data it can examine.
3. Predictive Analytics for Smarter Prioritization
The ability of AI to apply predictive analytics to identify which leads are most likely to convert is one of its most powerful aspects in lead scoring. AI can predict a lead's probability of becoming a paying client by training machine learning models on historical data (such as previous sales, customer interactions, and conversion rates).
AI can assess factors like:
- Lead activity: How often a lead interacts with the sales staff, attends demos, or engages with content.
- Lead profile: The degree to which a lead's business and title match those of your top clients.
- Past interactions: if a lead already showed interest in your offering by downloading a brochure or requesting a demo.
Instead of wasting time on leads that have little chance of closing, sales teams may focus their efforts on those that are most likely to convert thanks to these projections.
Why it matters: Sales teams may make the best use of their time and resources by prioritizing leads with the highest conversion rate with the help of predictive analytics.
4. Continuous Learning and Adaptation
AI-powered lead scoring algorithms are always learning and changing in response to fresh information and results. For example, the AI system can adapt its algorithms to identify trends in a lead's behavior, profile, and interactions after a sales team closes a deal with that lead. Over time, the lead scoring system becomes more precise and effective thanks to this ongoing learning process.
For instance, the AI system will start to prioritize similar leads in the future if it discovers that some leads from a particular industry or region have a higher chance of converting.
Why it matters: Over time, better decision-making results from lead scoring that is increasingly more accurate because of AI's capacity to learn from new data.
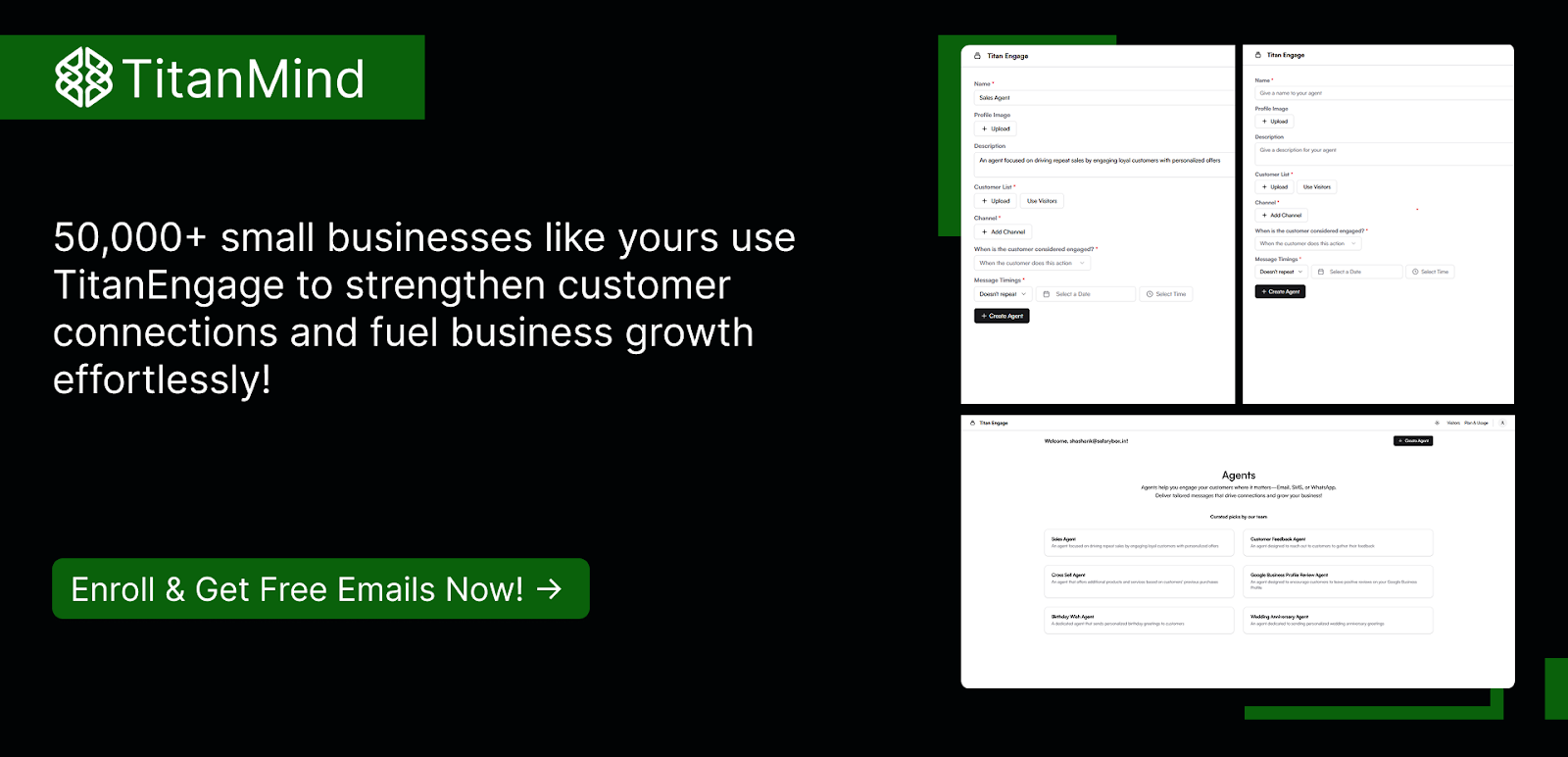
5. Real-Time Lead Scoring
AI-powered systems can offer real-time lead scoring, compared with traditional approaches that update lead scores manually or on a periodic basis. This implies that a lead's lead score is automatically updated whenever they connect with your brand in any way, such as by visiting your website or opening an email.
Sales teams can take rapid action on new leads thanks to real-time lead scoring, which also offers them the most recent information on which leads need attention. For example, if a lead spends a lot of time on your price page, it may result in a high lead score instantly, notifying the sales staff to follow up right away.
Why it matters: Sales teams can respond quickly and strategically with real-time insights, ensuring that no important lead is lost because of delayed data.
6. Eliminating Bias and Enhancing Objectivity
As human salespeople may rank leads according to subjective assessments or personal preferences, traditional lead scoring frequently suffers from unfairness. However, AI eliminates human judgment from the equation. AI-based systems base their objective lead prioritization judgments on data and patterns.
Also, AI systems can be configured to ignore unnecessary components like preconceptions or personal preferences in favor of measurements and actions that are actually important for conversions.
Why it matters: By ensuring that lead scoring is only based on data-driven insights, artificial intelligence (AI) improves objectivity and reduces the possibility of missed opportunities brought on by bias or human error.
7. Improved Collaboration Between Sales and Marketing Teams
AI-powered lead scoring helps close the gap between sales and marketing teams. Both teams can work together more successfully and have access to the same insights because of AI. Based on data insights, marketing teams may provide high-quality leads, and sales teams can utilize AI to further qualify and rank those leads.
Throughout the sales funnel, improved handoffs, seamless transitions, and more effective teamwork result from this alignment.
Why it matters: Better lead nurturing, qualification, and conversion are guaranteed when the sales and marketing teams work together more effectively.
Conclusion: Empowering Sales Teams with AI
Sales teams' strategies for prioritizing and scoring leads are being completely transformed by AI. AI allows sales teams to work smarter, not harder, by automating data collecting, integrating various data points, and employing predictive analytics. Sales teams are further guaranteed to concentrate their efforts on the most promising leads through real-time updates, ongoing learning, and objective decision-making, which eventually raises the possibility of conversion and enhances overall sales performance.
AI-powered lead scoring and prioritization will become an essential tool for sales teams as companies grow and lead management becomes more complex. Businesses may maximize sales efforts, optimize workflows, and make data-driven decisions that accelerate growth and success in a market that is becoming more and more competitive by embracing AI.